Deep Learning based Buy Predictions with Sequence Models
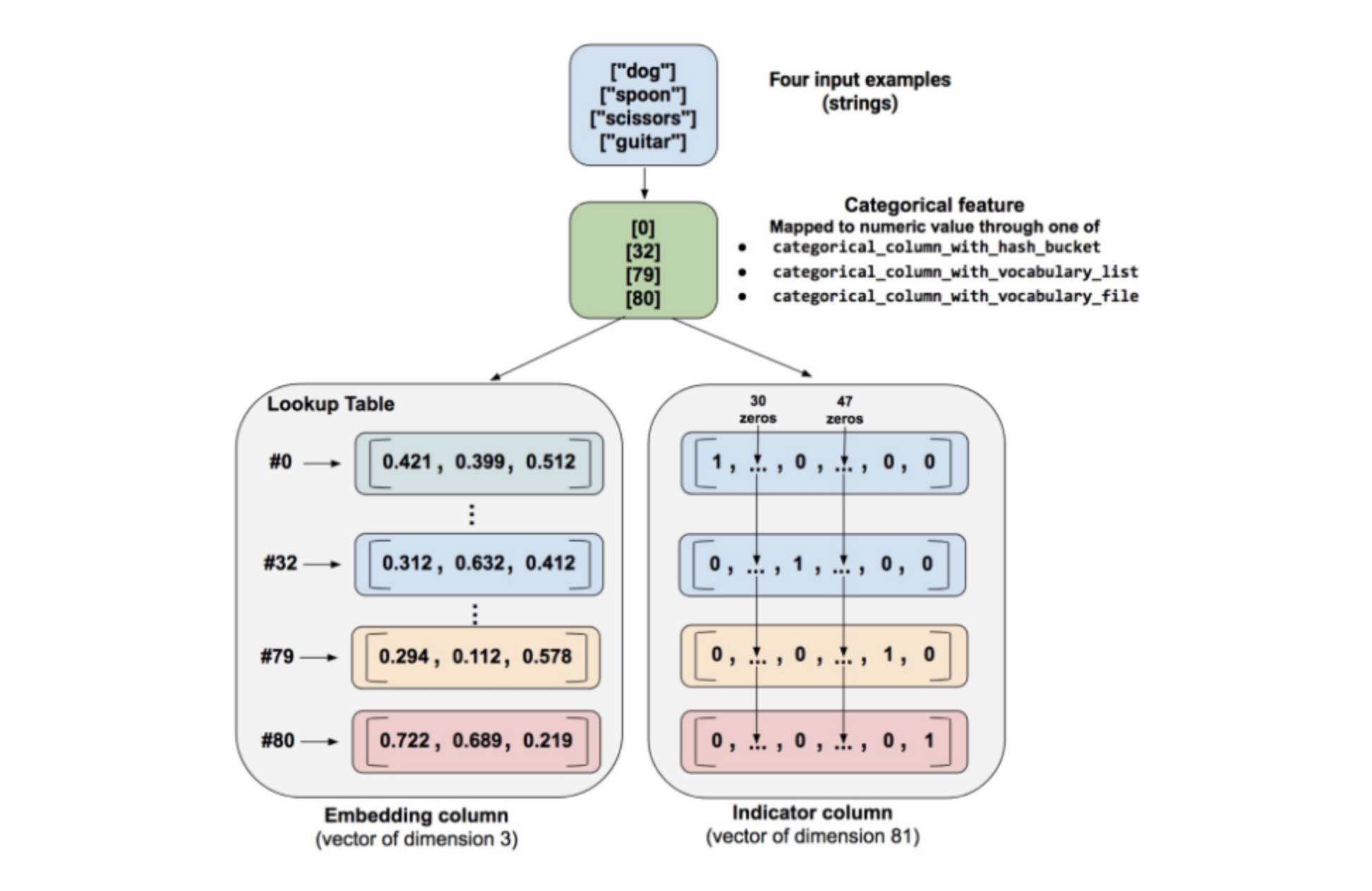
Identifying whether a clicked item in an e-commerce session will end in a purchase is a current research inquiry. This task was also set at the RecSys Challenge in 2015. The competition was won with a two-stage approach by Romov and Sokolov. They first recognized the buyers with the help of session data and then determined the purchased items on the recognized purchase sessions. However, in the features of their models, there is no information about the exact click sequences of the items that were viewed in a session. Therefore, in this paper, I examine the question of whether the purchase of products depends on the click stream of the items that are viewed in a session. For this purpose, I train sequence models on the item clicks of the sessions with purchases to check whether they can help predict purchased items. Here, I use the two sequence models of sequence classification and sequence labeling. I combine my sequence models with those of Romov and Sokolov to determine if their approach improves with sequence information. This research demonstrates that buying an item depends on the click order of the items that are viewed and can improve the predictive results of a feature-based approach, such as that of Romov and Sokolov. In addition, the research illustrates that data preparation is less time-consuming for this approach than for a feature based approach.
Master Thesis
Deep Learning based Buy Predictions with Sequence Models
Tasmin Herman, 2019, Link