Towards German Abstractive Text Summarization using Deep Learning
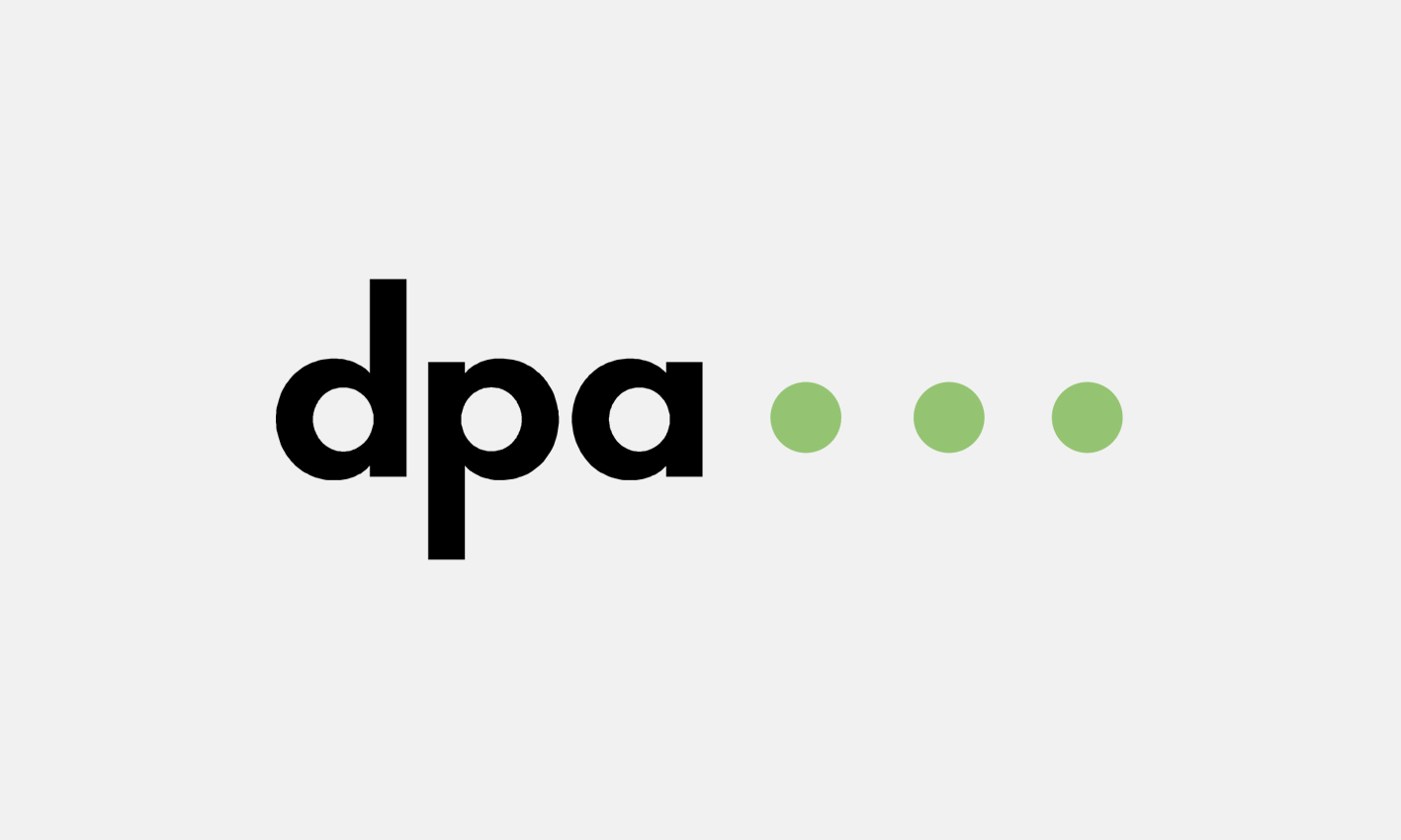
Text summarization is an established sequence learning problem divided into extractive and abstractive models. While extractive models learn to only rank words and sentences, abstractive models learn to generate language as well. The great success of deep learning algorithms on sequence learning tasks led to an increase in sequence to sequence learning algorithms with an attention mechanism. At the same time, the research area around transfer learning, transferring knowledge different domains, languages and tasks, increased over the past years. Word embeddings like GloVe or Word2Vec, are still useful for practical purposes, but were overtaken by a new generation of language models. In this thesis we explore two of the most prominent language models named ELMo and BERT, applying them to the extractive summarization task. We contribute a new ensemble model between abstractive and extractive summarization achieving, a new state-of-the-art on the English CNN/DM dataset. Instead of only working with an academic English dataset, we introduce a new dataset in German from the Deutsche Presse Agentur (DPA). This poses a challenge since real world datasets in German have less available resources through pretrained language models and inhibit more noise. We establish several abstractive and extractive summarization baselines.
Master Thesis
Towards German Abstractive Text Summarization using Deep Learning
Matthias Nitsche, 2019, Link